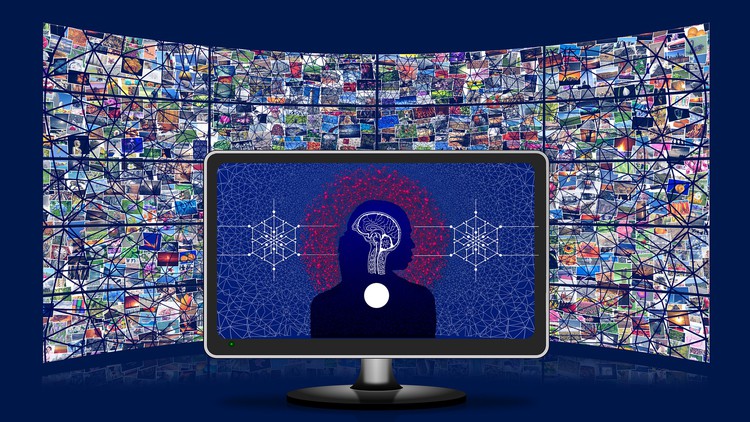
Description
This course is designed to teach you how to build a video classification model using Keras and TensorFlow, with a focus on action recognition. Video classification has numerous applications, from surveillance to entertainment, making it an essential skill in today’s data-driven world. Through this course, you will learn how to extract features from video frames using pre-trained convolutional neural networks, preprocess the video data for use in a custom prediction loop, and train a Transformer-based classification model using Keras.
By the end of this course, you will be able to build your own video classification model and apply it to various real-world scenarios. You will gain a deep understanding of deep learning techniques, including feature extraction, preprocessing, and training with Keras and TensorFlow. Additionally, you will learn how to optimize and fine-tune your model for better accuracy.
This course is suitable for anyone interested in deep learning and video classification, including data scientists, machine learning engineers, and computer vision experts. The demand for professionals skilled in deep learning and video classification is increasing rapidly in the industry, and this course will equip you with the necessary skills to stay ahead of the competition.
Join us today and take the first step towards becoming an expert in video classification using Keras and TensorFlow!
Who this course is for:
- Python developers interested in machine learning and video classification
- Data scientists looking to expand their knowledge in computer vision and deep learning
- Students or professionals in the field of computer science and engineering interested in developing and deploying video classification models
- Anyone interested in learning how to implement a state-of-the-art video classification model using Keras and TensorFlow.
Requirements
- Basic knowledge of Python Programming
Last Updated 3/2023
Download Links
Direct Download
Python-based Video Classification with Deep Learning.zip (515.5 MB) | Mirror